
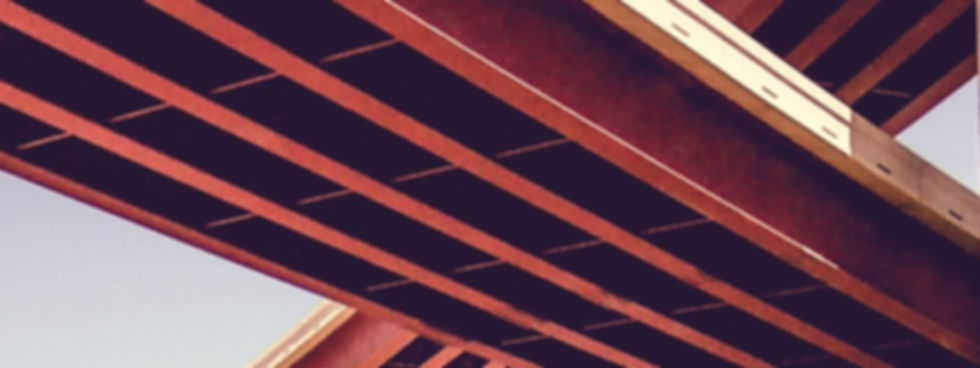
Research
Ground motion intensity measure selection for high-confidence seismic risk assessment
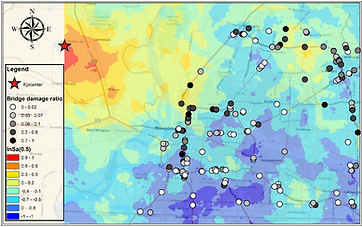
Ground motion intensity measures (IMs) serve as a critical link from seismic hazard to structural seismic demands (i.e., responses). By leveraging differential entropy from information theory to quantify the uncertainties of multivariate random variables, we proposed a novel entropy-based IM selection approach to holistically constrain the uncertainty contributions from both seismic hazard and stochastic structural responses to achieve more consistent and confident seismic risk assessment (SRA). This IM selection methodology was examined both in site-specific SRA and regional-level SRA.
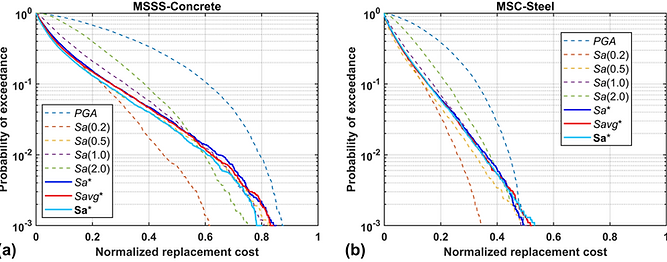
Multivariate return period based ground motion record selection
We proposed a multivariate return period (MRP)-based ground motion selection methodology to identify hazard-consistent ground motions by holistically incorporating the joint rate of exceedance of vector conditioning intensity measures (IMs). This approach features several favorable properties that allow more objective ground motion selection for intensity-based
assessment, including: (1) moderate target spectra intensity, and moderately low target spectra standard deviation across a wide range of IMs; (2) superior convergence with the increase of the conditioning vector IM dimension, and ability to approximate higher-dimensional hazard consistency with lower dimensional conditioning IMs; and (3) capability to realistically incorporate multiple causal earthquakes owing to the implementation of multivariate Gaussian mixture distribution in simulating the response spectra. We further proposed a refined ground motion selection approach with significantly improved computational efficiency and we implemented the MRP-based ground motion selection into seismic risk assessment. The proposed ground motion selection method can lead to unbiased risk estimates while robustly accomodating structural systems sensitive to multiple IMs.

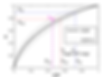
Parameterized multivariate seismic surrogate demand modeling of complex civil structure and infrastructure systems
Probabilistic seismic risk assessment of complex multi-response structural systems requires accurate estimations of multiple engineering demand parameters (EDPs) that are related to different failure modes. One conventional way is developing multiple separate univariate seismic demand models for each of the EDPs. However, such an approach lacks sufficient consideration of the correlations among different EDPs and also requires repetitive model tuning and training. Another alternative, which has not yet been extensively studied, is to develop a single multivariate surrogate demand model (MvSDM) that is able to jointly provide the estimates for all the EDPs. To facilitate more accurate and realistic seismic demand modeling of multi-response structural systems, the present study conducts a comprehensive investigation of different MvSDMs, which are constituted by a systematic trend model that characterizes the mean response hypersurface, and a covariance model that quantifies the correlated model errors. Three types of systematic trend models including multivariate linear regression (MvLR), partial least squares regression (PLSR) and artificial neural network (ANN); along with three model error covariance estimation methods including joint probabilistic demand model (JPSDM) method, sample regression residual covariance (SCOV) method and multi-output Gaussian process regression (MOGP), are considered. The influence of trend model and error covariance model selection on seismic demand and fragility modeling is then studied based on a typical three-span concrete-girder highway bridge structure in the Central and Southeastern US. The results suggest that kernel PLSR and ANN as the systematic trend models, and the SCOV method as the error covariance model are promising alternatives that can contribute to accurate and reliable multivariate surrogate
demand modeling yet with good computational efficiency.
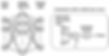

Metamodel-based state-dependent fragility modeling for Markovian sequential seismic damage assessment
Accurate characterization of seismic damage accumulation is crucial to reliable seismic risk assessment. Emerging research efforts have been devoted to state-dependent fragility modeling considering the impact of sequential earthquake excitations so as to better inform the management of structure and infrastructure assets. However, the current state-dependent fragility models largely rely on simple functional forms conditioned on only a scalar ground motion intensity measure (IM); fragility models are separately developed for different state transition scenarios, without any joint modeling mechanism to preserve the multivariate nature among the different damage states; and the lack of parameterization further hinders these models from offering pertinent fragility estimates for structural portfolios with uncertain structural parameters. By leveraging advanced ground motion selection and machine learning techniques, this study proposes a novel metamodel-based state-dependent fragility modeling approach that offers superior parameterization and damage classification capability and can be seamlessly integrated into the Markovian sequential seismic damage assessment (SSDA) framework. The proposed fragility modeling approach features a systematic sequential dynamic analysis procedure that leverages efficient experimental design and hazard-consistent ground motion selection to sufficiently cover the feature space, and an artificial neural network (ANN) classification model conditioned on one-hot encoded initial damage states along with other earthquake and structure-related predictors. Based on generic inelastic singledegree-of-freedom (SDOF) systems with varying structural configurations, damage state classification performance of the proposed fragility model is thoroughly evaluated, and its applicability within the Markovian SSDA framework is elaborated and discussed.

Experimental and numerical study of bolted connections for pultruded glass fiber-reinforced polymer bridge decks
To numerically investigate failure behavior of pultruded fiber reinforced polymer (PFRP) bolted joints, a progressive damage analysis (PDA) material model integrating nonlinear shear response, Hashin-type failure criteria and strain-based continuous degradation rules was proposed in the present study. The PDA model was implemented via user subroutine VUMAT, detailed 3D finite element models were established in Abaqus/Explicit. Experiments were conducted to validate the proposed PDA model. Result shows that explicit finite element method is computational efficient and can effectively avoid convergence problem. The predicted joint loading response correlates well with the test result. The FE model can accurately capture various failure modes including bearing, shear-out and net-tension and reveal failure propagation and damage mechanism of PFRP bolted joints. Emergence of first fiber compression failure is found to be a good indicator to identify the load at which the load–displacement response becomes nonlinear
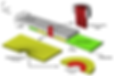
